Single Wheat Seed Volume Measurements as Estimated from Orthogonal Top and Side Images.
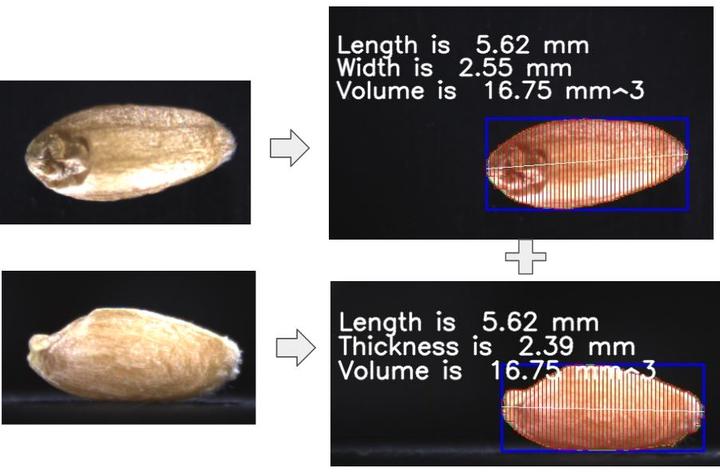
Computer vision and pattern recognition deal with how computers can be made to gain a high-level understanding of images or videos. The field of machine learning has grown rapidly over the past few years and practitioners can inject machine learning to agriculture applications. The affordable consumer-grade cameras have brought about a profound advancement of image processing methods. The elliptical cylinder model performs great on the variety ofwheat seed samples including milo and whea.t. For the number of slices selection, the error rate is below 3% on selected reference objects when we choose N=50 number of slices or above. Due to the lack of the seed image processing framework for volume measurement in the agriculture field, we proposed a framework that can calculate the seed volume by measuring its cross-section using two cameras to gather image information. First, we set up a turntable with two cameras: the main view and side view. Next, we turn on the seed feeder and turntable, and our framework captures pictures for one single seed dynamically. Then, our model makes image analysis on two images of one seed and displays the result in real-time through the framework. Combining knowledge of seed image analysis, our proposed framework performs state of the art imaging processing model on seed analysis and achieves rapid measurement of seed volume. The results of proposed volume measurements using wheat seed samples are compared with several image-based volume measurements, and the impact of different experiment design is also analyzed. This framework significantly improves the efficiency in the field of seed property analysis for the researchers.